AI identified these 5 types of heart failure in new study: ‘Interesting to differentiate’
“Heart failure” is a catch-all term used to describe any condition in which the organ doesn’t work as it’s supposed to — but one person’s experience with the disease can be very different from someone else’s.
Researchers from the University College London (UCL) recently used machine learning — a type of artificial intelligence — to pinpoint five distinct types of heart failure, with the goal of predicting the prognosis for the different kinds.
“We sought to improve how we classify heart failure, with the aim of better understanding the likely course of disease and communicating this to patients,” said lead author Professor Amitava Banerjee from UCL in a press release announcing the study.
HEART DISEASE, THE SILENT KILLER: STUDY SHOWS IT CAN STRIKE WITHOUT SYMPTOMS
“Currently, how the disease progresses is hard to predict for individual patients,” he also said. “Some people will be stable for many years, while others get worse quickly.”
The five types of heart failure identified were early onset, late onset, atrial fibrillation (which causes an irregular heart rhythm), metabolic (linked to obesity but with a low rate of cardiovascular disease) and cardiometabolic (linked to obesity and cardiovascular disease), according to a press release on UCL’s website.
“The five types of heart failure were on the basis of common risk factors, such as age at onset of heart failure, history of cardiac disease, history of cardiac risk factors such as diabetes and obesity, or atrial fibrillation (the commonest heart rhythm problem),” explained Banerjee in a statement to Fox News Digital.
For the study, published in the journal Lancet Digital Health, the researchers analyzed data from more than 300,000 U.K. adults aged 30 and older who had experienced heart failure over a 20-year period.
HEART DISEASE RISK COULD BE AFFECTED BY ONE SURPRISING FACTOR, NEW STUDY FINDS
“Four methods of machine learning were used to cluster individuals with heart failure in electronic health data by their baseline characteristics,” said Banerjee. “The method and the number of clusters that ‘fit’ best to the data were selected.”
For each type of heart failure, the researchers determined the likelihood of the person dying within a year of diagnosis. The prognosis varied widely for the five subtypes, they found.
The five-year mortality risk was 20% for early onset, 46% for late onset, 61% for atrial fibrillation-related, 11% for metabolic and 37% for cardiometabolic, according to the press release.
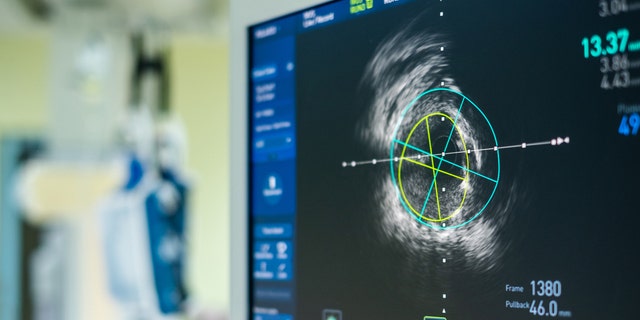
For health professionals, Banerjee recommends that they ask their heart failure patients about common risk factors to help them understand the subtype they have.
“Researchers also need to test how usable, generalizable and acceptable these subtypes defined in our study are in clinical practice,” he added.
“They should also consider whether studies such as ours, which use AI, can help inform a better understanding of disease processes and drug discovery.”
The research team also developed an app for physicians that would enable them to determine which subtype of heart failure a patient has — with the goal of better predicting risk and keeping patients informed.
AI AND HEART HEALTH: MACHINES DO A BETTER JOB OF READING ULTRASOUNDS THAN SONOGRAPHERS DO, SAYS STUDY
Dr. Ernst von Schwarz, a triple board-certified clinical and academic cardiologist at UCLA in California, reviewed the results of UCL’s study.
“For clinicians, it is interesting to differentiate heart failure according to prognosis, which usually is not done in the clinical setting,” he told Fox News Digital. “Heart failure is generally seen as an incurable, chronic, progressive disease with poor long-term outcomes.”
“Heart failure is generally seen as an incurable, chronic, progressive disease with poor long-term outcomes.”
“Studies like this might help clinicians make a more appropriate risk assessment according to the etiology of heart failure,” von Schwarz added.
In particular, the very high mortality rate for atrial fibrillation-induced heart failure highlights the importance of aggressively managing this common arrhythmia, he said.
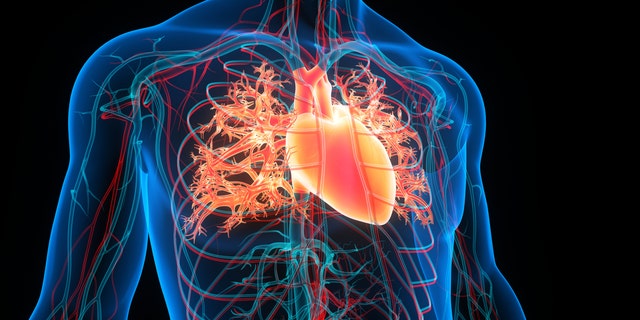
The mortality predictions for the five subtypes are “by far the most interesting part of this data,” according to Dr. Matthew Goldstein, a physician at Cardiology Consultants of Philadelphia, who also reviewed the study findings.
“This may help us guide who is at risk for dying suddenly, and thus, who needs protection with a defibrillator and who does not,” he added.
AI shows promise, but limitations remain
While Goldstein recognizes that AI is becoming more common in general, he believes its application is medicine has shown “somewhat less success.”
He told Fox News Digital, “It is, however, good at looking for patterns that are too complicated for the human mind to see.”
AI TECHNOLOGY CATCHES CANCER BEFORE SYMPTOMS WITH EZRA, A FULL-BODY MRI SCANNER
“Some of the more common utilizations are automatic readings of radiology studies to make sure that nothing is missed and emerging use in EKG interpretation to suggest underlying pathology,” he added.
In terms of using AI to classify heart failure, Goldstein noted that this is only a retrospective study and will need to be proven for future cases in order to be truly useful.
Looking ahead
The main limitation of the new study was that the researchers didn’t have access to any imaging data, which is most commonly used to diagnose and predict risk in heart failure.
“However, imaging markers alone do not predict mortality and other outcomes,” Banerjee said.
“The fact that we were able to use routinely collected data without this imaging data to predict subtypes and outcomes relatively well suggests that the imaging biomarkers alone may not be the best way to characterize and study heart failure at population scale.”
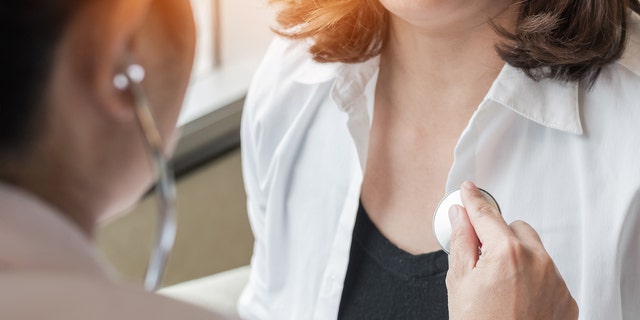
The next step, Banerjee said, is to determine whether classifying various heart failures can make a practical difference to patients — “whether it improves predictions of risk and the quality of information clinicians provide, and whether it changes patients’ treatment.”
CLICK HERE TO SIGN UP FOR OUR HEALTH NEWSLETTER
Cost-effectiveness is another consideration, he added.
The UCL research team previously used similar methods to identify subtypes in chronic kidney disease.
Looking ahead, Banerjee expects that machine learning will be used to analyze many types of routinely collected medical data and to identify subtypes of different diseases.
Read the full article Here