AI may have an ‘eye’ on growing babies: Could predict premature birth as early as 31 weeks
About 10% of all infants born in the U.S. in 2021 were preterm — which means they were delivered earlier than 37 weeks of pregnancy, per the Centers for Disease Control and Prevention (CDC).
Preterm births also make up about 16% of infant deaths.
Now, researchers from Washington University in St. Louis, Missouri, are looking to improve those odds through the use of artificial intelligence.
They developed a deep learning model that can predict preterm births by analyzing electrical activity in the woman’s uterus during pregnancy — then they tested the model in a study that was published in the medical journal PLOS One.
AI TOOL HELPS DOCTORS MAKE SENSE OF CHAOTIC PATIENT DATA AND IDENTIFY DISEASES: ‘MORE MEANINGFUL’ INTERACTION
“The key takeaway is that it is possible to take data as early as the 31st week and predict preterm birth up to the 37th week” — which surprised the researchers, Arye Nehorai, PhD, professor of electrical engineering at Washington University in St. Louis, told Fox News Digital.
“The AI/deep learning automatically learned the most informative features from the data that are relevant to the prediction of preterm birth,” he added.
Additionally, the findings indicate that preterm birth is an abnormal physiological condition, not just a pregnancy that happened to end early, Nehorai said.
During the study, the researchers performed electrohysterograms (EHGs), which use electrodes on the abdomen to record electrical activity in the uterus.
They took recordings of these electrical currents from 159 pregnant women who were at least 26 weeks along and “trained” the AI model on that data.
RUPTURED BRAIN ANEURYSM LANDS SOCIAL MEDIA INFLUENCER IN MEDICALLY INDUCED COMA AFTER EMERGENCY C-SECTION
They combined this data with medical information such as the woman’s age and weight, the fetal weight and any bleeding experienced in the first or second trimester.
Nearly 19% of the women in the study delivered preterm. In theory, the data from those women could be used as a benchmark for predicting premature birth.
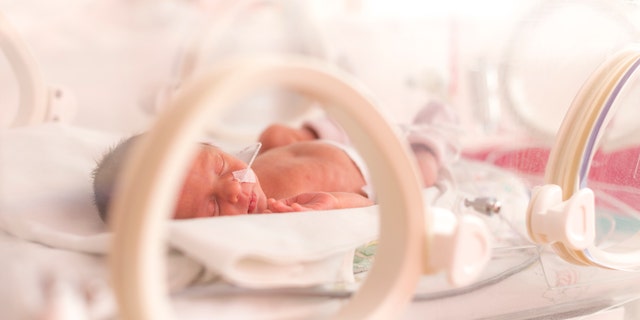
“The advantage of our approach is that is inexpensive to build,” said Nehorai of the new research. “Our model was effective in prediction with shorter EHG recordings, which could make the model easier to use, more cost-effective in a clinical setting and possibly usable in a home setting.”
Looking ahead, the researchers believe that this method should be adopted by hospitals and obstetricians as part of women’s regular pregnancy check-ups. It would then allow pregnant women to seek care and make lifestyle changes to protect the health of their baby as needed.
“Our work contributes to the goal of using EHG measuring devices for accurately predicting preterm birth.”
“A device dedicated to implementing our method should be built for this purpose,” said Nehorai.
It is hard to say how long it might be before this kind of test becomes widely available, the researchers said.
“There are some EHG measuring devices already in the market — however, it has been challenging to predict preterm births from the EHG data,” said Uri Goldsztejn, a PhD candidate in the department of biomedical engineering working under the supervision of Professor Nehorai at Washington University.
NEW AI ‘CANCER CHATBOT’ PROVIDES PATIENTS AND FAMILIES WITH 24/7 SUPPORT: ‘EMPATHETIC APPROACH’
“Our work contributes to the goal of using EHG measuring devices for accurately predicting preterm birth,” he told Fox News Digital.
EHG measurements usually take between 30 and 60 minutes, with additional time required for setting the device on the mother’s abdomen, noted Goldsztejn.
“We showed that predictions could be made based on shorter EHG measurements, of under five minutes, without reducing the prediction accuracy too much,” he told Fox News Digital. “This finding is significant, since the prolonged duration of EHG measurements is an important limitation for its adoption in clinical settings.”
‘Promise’ of deep learning — but caveats
Dr. Suzy Lipinski, a board-certified OB/GYN at Pediatrix Medical Group in Denver, Colorado, was not involved in the study but shared her input on whether the deep learning technology could help solve the problem of preterm birth in the U.S.
“Being able to predict who is at risk before they present in labor would be highly beneficial,” Lipinski told Fox News Digital. “The use of a deep learning model seems to hold promise; however, this study has a relatively small number of patients, so it cannot be determined how applicable this is to a larger population.”
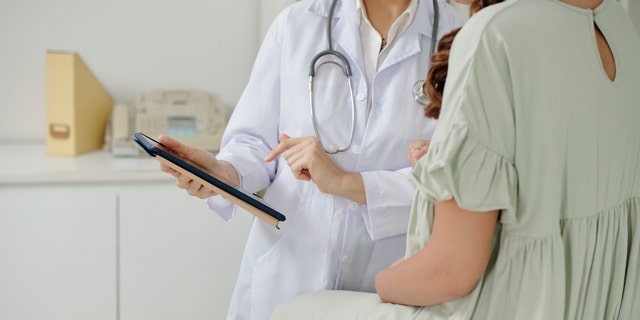
“Prior studies using AI have not shown great reliability, so this will need more studies and larger patient populations before we start using this method,” she added.
Another potential limitation is that very few places use EHG measurements, the doctor pointed out.
“The standard in most hospitals and offices is to use a tocodynamometer, which measures pressure, not electrical,” she explained.
OZEMPIC, WEGOVY AND PREGNANCY RISK: WHAT YOU NEED TO KNOW ABOUT THE ISSUE
If EHG becomes the way to evaluate for preterm birth, then hospitals, birth centers and offices would all have to purchase new equipment, which could delay adoption in low-resource areas such as rural and inner cities, Lipinski said.
“A higher rate of preterm birth in this study than the national average also raises questions about the applicability,” she told Fox News Digital. “No demographics were given about the patient population, so there is no way to see how it reflects the population of the entire country.”
“Being able to predict who is at risk before they present in labor would be highly beneficial.”
There is also the chance of false positives, Lipinski pointed out.
“While this method does predict better than our current methods, there are still many patients that will be identified as at-risk that may not have a preterm birth,” she said. “This false positive result will cause a large burden of stress on the patient, as well as increased utilization of health care resources.”
If and when this becomes the new standard of care, Lipinski said, there would need to be improved treatments for preterm labor.
“Our issues with preterm birth are two-fold: We have poor prediction, but also poor prevention options after 26 weeks,” she added.
Researchers share study’s main limitations
The study has two main limitations, according to Goldsztejn.
“First, we developed our work using around 160 samples from two public datasets,” he said. “Although this amount of data was sufficient for our initial investigation, a far larger dataset would be required for developing and validating a medical product.”
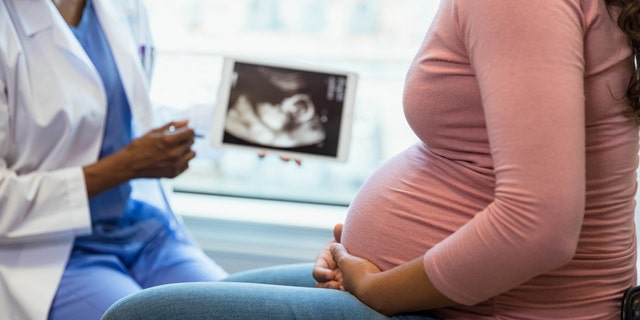
The second limitation comes from the nature of deep learning, which can produce accurate results but is usually hard to interpret, Goldsztejn said.
“In other words, it is challenging to understand how the algorithm makes predictions,” he explained.
CLICK HERE TO SIGN UP FOR OUR HEALTH NEWSLETTER
In a discussion of the findings in the medical journal, the authors noted that “although machine learning algorithms can contribute to improving health care and much research is yielding advances in this field, important challenges remain.”
“A far larger dataset would be required for developing and validating a medical product.”
Among those challenges: It can be difficult to identify the causes behind the algorithm’s predictions, the researchers wrote.
“In our case, although our predictions could influence pregnancy management, our predictions would need to be supplemented with additional medical examinations to determine which therapies are more likely to reduce the risk of preterm birth and improve its outcomes,” the researchers also said.
Read the full article Here